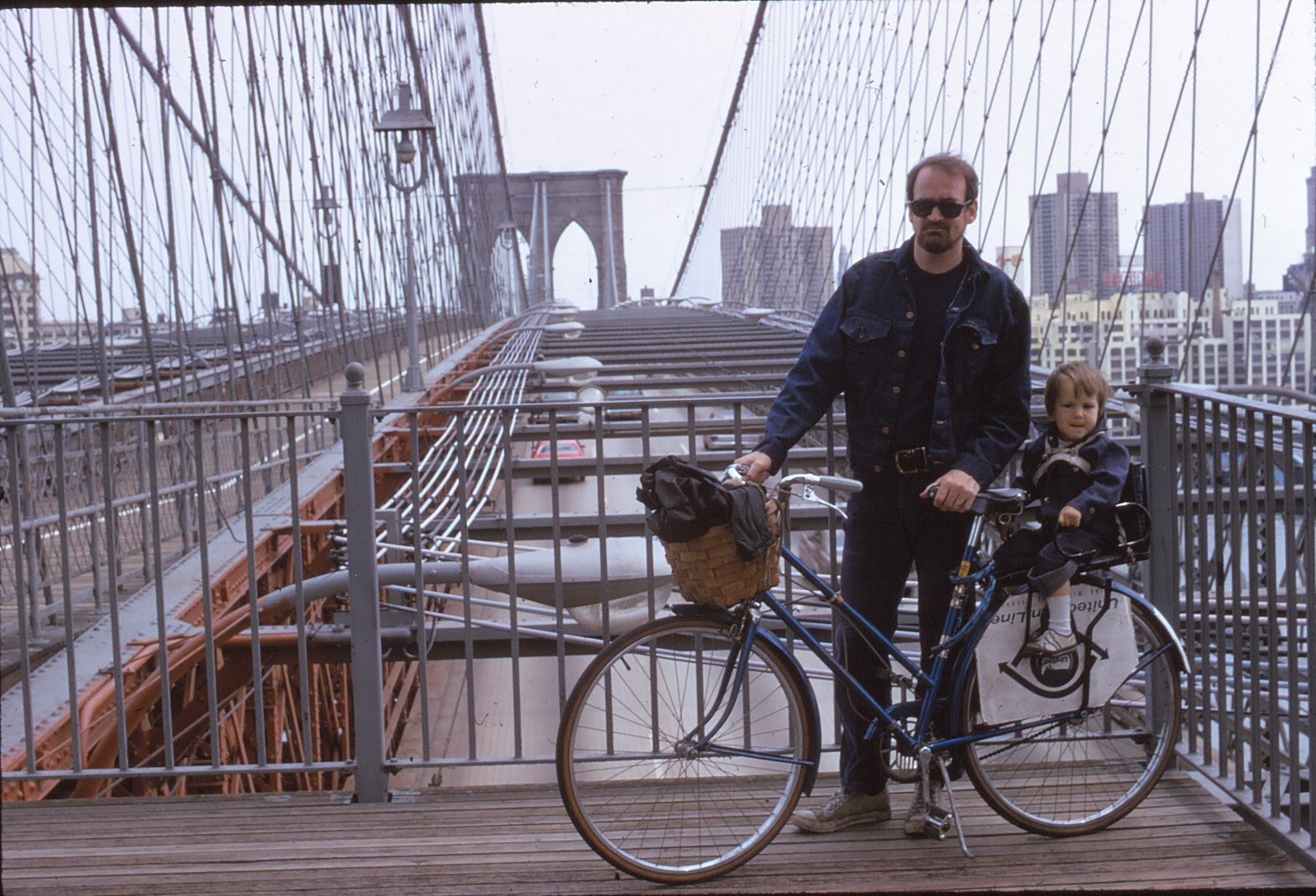
the data will out.
Over a month ago (how time flies), we were asked to pull together three ideas for a “Capstone” project for my General Assembly Data Science Immersive class. I was not feeling particularly inspired at the time and searched for data sets within my non-academic interests. I proposed three datasets (not projects): AirBnB in Paris, NYC Tree Census, and bike share data. (I can’t say I’ve ridden a bike share, but I have rented bikes in foreign cities including Amsterdam, my husband uses the local bike share quite frequently, and I’m intrigued by the idea.)
As I dug further, I found that the datasets for AirBnB and NYC trees are quite “large”, and the AirBnB set lacked rental data. The questions that came to mind regarding these datasets were not going to have any “answers”. So I dove into the bike share data. This was a dataset I could take a bite out of and chew on. And then after finding data from other bike shares across the country? Chew, chew, chew.
But then that unwelcome “Eureka” moment. Lots of data. Interesting plots. But nothing of interest to model in terms of correlations, predictions, etc.
Low moment in my Capstone project. I was done for. What now?
No throwing in the towel.
I refused to quit. The data in hand was not enough. What could I add to make it interesting?
Common sense prevails (this could possibly be considered domain knowledge, but here? common sense.). When do you ride a bike? When it is rainy and cold? Or when it is a beautiful day? Taking it further, would you go for a ride at 7pm if it is light out? And would you if night has settled in? Or do you use it when weather is bad and you have to get to work and that’s the best option?
Time to scrape weather data and daylight data and get to work building features!
My first dataset was interesting, but lacked the background to tell the story. By adding in weather data and daylight data (though this might also be highly correlated with temperature and summer vacations), I was able to generate interesting results for most cities. Not perfect correlations, but definitely on the trail to something interesting….
Los Angeles still refuses to fall into line and let me predict ridership rates. I hope to add in air quality data (or other domain knowledge!?!) for modeling LA, but I am still searching.
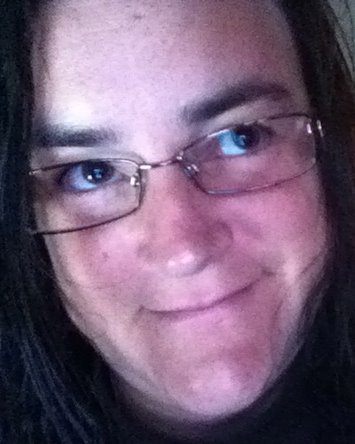
GBK Gwyneth
Leave a Reply